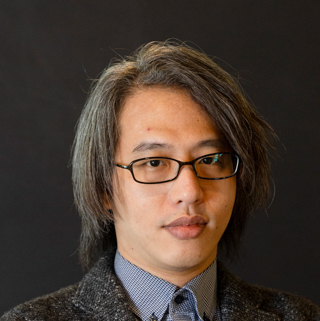
Wei-Hsiang
Liao
Publications
We introduce the Robust Audio Watermarking Benchmark (RAW-Bench), a benchmark for evaluating deep learning-based audio watermarking methods with standardized and systematic comparisons. To simulate real-world usage, we introduce a comprehensive audio attack pipeline with var…
Recent state-of-the-art neural audio compression models have progressively adopted residual vector quantization (RVQ). Despite this success, these models employ a fixed number of codebooks per frame, which can be suboptimal in terms of rate-distortion tradeoff, particularly …
Music timbre transfer is a challenging task that involves modifying the timbral characteristics of an audio signal while preserving its melodic structure. In this paper, we propose a novel method based on dual diffusion bridges, trained using the CocoChorales Dataset, which …
Recent state-of-the-art neural audio compression models have progressively adopted residual vector quantization (RVQ). Despite this success, these models employ a fixed number of codebooks per frame, which can be suboptimal in terms of rate-distortion tradeoff, particularly …
Existing work on pitch and timbre disentanglement has been mostly focused on single-instrument music audio, excluding the cases where multiple instruments are presented. To fill the gap, we propose DisMix, a generative framework in which the pitch and timbre representations …
This paper presents a novel approach to deter unauthorized deepfakes and enable user tracking in generative models, even when the user has full access to the model parameters, by integrating key-based model authentication with watermarking techniques. Our method involves pro…
Controllable generation through Stable Diffusion (SD) fine-tuning aims to improve fidelity, safety, and alignment with human guidance. Existing reinforcement learning from human feedback methods usually rely on predefined heuristic reward functions or pretrained reward model…
To accelerate sampling, diffusion models (DMs) are often distilled into generators that directly map noise to data in a single step. In this approach, the resolution of the generator is fundamentally limited by that of the teacher DM. To overcome this limitation, we propose …
Contrastive cross-modal models such as CLIP and CLAP aid various vision-language (VL) and audio-language (AL) tasks. However, there has been limited investigation of and improvement in their language encoder, which is the central component of encoding natural language descri…
Recent advancements in music generation are raising multiple concerns about the implications of AI in creative music processes, current business models and impacts related to intellectual property management. A relevant challenge is the potential replication and plagiarism o…
In the realm of audio watermarking, it is challenging to simultaneously encode imperceptible messages while enhancing the message capacity and robustness. Although recent advancements in deep learning-based methods bolster the message capacity and robustness over traditional…
Music mixing is compositional -- experts combine multiple audio processors to achieve a cohesive mix from dry source tracks. We propose a method to reverse engineer this process from the input and output audio. First, we create a mixing console that applies all available pro…
Vector quantization (VQ) is a technique to deterministically learn features with discrete codebook representations. It is commonly performed with a variational autoencoding model, VQ-VAE, which can be further extended to hierarchical structures for making high-fidelity recon…
Restoring degraded music signals is essential to enhance audio quality for downstream music manipulation. Recent diffusion-based music restoration methods have demonstrated impressive performance, and among them, diffusion posterior sampling (DPS) stands out given its intrin…
In recent years, research on music transcription has focused mainly on architecture design and instrument-specific data acquisition. With the lack of availability of diverse datasets, progress is often limited to solo-instrument tasks such as piano transcription. Several wor…
Despite the recent advancements, conditional image generation still faces challenges of cost, generalizability, and the need for task-specific training. In this paper, we propose Manifold Preserving Guided Diffusion (MPGD), a training-free conditional generation framework th…
Consistency Models (CM) (Song et al., 2023) accelerate score-based diffusion model sampling at the cost of sample quality but lack a natural way to trade-off quality for speed. To address this limitation, we propose Consistency Trajectory Model (CTM), a generalization encomp…
Restoring degraded music signals is essential to enhance audio quality for downstream music manipulation. Recent diffusion-based music restoration methods have demonstrated impressive performance, and among them, diffusion posterior sampling (DPS) stands out given its intrin…
In recent years, research on music transcription has focused mainly on architecture design and instrument-specific data acquisition. With the lack of availability of diverse datasets, progress is often limited to solo-instrument tasks such as piano transcription. Several wor…
Taking long-term spectral and temporal dependencies into account is essential for automatic piano transcription. This is especially helpful when determining the precise onset and offset for each note in the polyphonic piano content. In this case, we may rely on the capabilit…
We propose an end-to-end music mixing style transfer system that converts the mixing style of an input multitrack to that of a reference song. This is achieved with an encoder pre-trained with a contrastive objective to extract only audio effects related information from a r…
One noted issue of vector-quantized variational autoencoder (VQ-VAE) is that the learned discrete representation uses only a fraction of the full capacity of the codebook, also known as codebook collapse. We hypothesize that the training scheme of VQ-VAE, which involves some…
JOIN US
Shape the Future of AI with Sony AI
We want to hear from those of you who have a strong desire
to shape the future of AI.