Beyond Skin Tone: A Multidimensional Measure of Apparent Skin Color
AI Ethics
September 21, 2023
Advancing Fairness in Computer Vision: A Multi-Dimensional Approach to Skin Color Analysis
In the ever-evolving landscape of artificial intelligence (AI) and computer vision, fairness is a principle that has gained substantial attention though less substantive solutions. The seminal paper "Gender Shades" by Buolamwini and Gebru1 opened many eyes to biases in gender classification systems, particularly those affecting individuals with darker skin tones.
Since then, fairness researchers and practitioners have sought to identify and mitigate these biases, often relying on the Fitzpatrick skin type classification as a standard measure for assessing skin color bias in computer vision systems. The Fitzpatrick scale, a valuable though blunt tool, offers an unidimensional view of skin tone, ranging from light to dark. However, as we delve deeper into the intricate world of human skin and its representation in AI models, we realize that this unidimensional approach may not capture the full spectrum of skin color complexities.
In this groundbreaking research paper, we take a significant step towards a more comprehensive understanding of apparent skin color in computer vision and a significant new solution for bias identification and assessment. We introduce a new dimension – the hue angle, which spans the range from red to yellow. By integrating this dimension into our analysis, we uncover where relevant and pernicious biases are being rendered invisible and find additional layers of bias related to apparent skin color within computer vision datasets and models.
We created fairness assessments of computer vision datasets and models to understand if there is discrimination towards specific skin color groups. In particular, the paper showcases several use cases, including quantifying skin color bias in existing face datasets, quantifying the skin color bias in Generative AI models and in models such as the Twitter (X) open-source image cropping model.
The project took inspiration from our personal experiences with invisibility, drawing from such diverse experiences as buying cosmetics and the work of artist Angelica Dass who took a portrait of thousands of Londoners and associated their skin color with Pantone colors to illustrate the diversity of human skin color. Her project Humanae was showcased in a TED talk and at London’s Migration Museum. Parallel to Angelica’s work, this research project strives for a more comprehensive representation of the skin color diversity to assess its effect on computer vision models.
“One of the things that particularly resonated with me was the fact that Dass mentioned that “nobody is ‘black,’ and absolutely nobody is ‘white’” and that very different ethnic backgrounds sometimes wind up with the exact same Pantone color. This is very similar to what we ended up finding when looking at skin color biases in image datasets and computer vision models,” noted Sony AI Ethics Research Scientist and author William Thong.
Alleviating an Entrenched Problem by Looking to Other Industries
In computer vision, skin tone bias is one of the most common forms of bias that developers check for (often as a proxy for race), but they have always only checked for this on the light-to-dark spectrum. Other industries, like the beauty industry, have begun to recognize the importance of having not only light-to-dark options but also considering skin undertones (warm-to-cool) in order to properly capture human skin tone diversity. But up until our paper, AI researchers have not considered this - perhaps in part because very few of them have had the experience of picking out makeup or matching a foundation shade to their skin color. This is problematic because it erases biases against East Asians, South Asians, Hispanics, Middle Eastern individuals, and others who might not neatly fit along the light-to-dark spectrum. Expanding the skin tone scale into two dimensions: light vs. dark and warm (yellow-leaning) vs. cool (red-leaning), can help to identify far more biases.
“For a long time, I struggled to find appropriate foundation matches in the US when shopping for make-up, until in recent years when brands started taking skin tone inclusivity seriously and introduced more shades in different undertones. As a result, I’ve long been aware of the importance of undertones and of the fact that as an East Asian individual, my undertone was historically not well-represented in US skin tone scales,” commented Alice Xiang, Lead Research Scientist, Sony AI and Global Head of AI Ethics for Sony Group Corporation. “When I started to work on fairness in computer vision, it struck me that we might be seeing a similar phenomenon, where the community was relying on a one-dimensional skin tone scale that reflected Caucasian skin tones but was not designed to reflect broader diversity. As a result, when I started the AI Ethics Lab at Sony AI, I wanted to investigate what the impact would be of expanding the dominant light-dark skin tone scale to include different skin undertones. Could we reflect more diversity and detect more biases? Our paper shows that we indeed can.”
In recent years, there has been growing awareness of the limitations of computer vision models to be biased against under-represented groups. It is thus critical to develop fairness tools that can help assess potential biases and document them in datasheets and model cards. In the context of this paper, we are interested in developing a fairness tool to better assess and quantify biases related to skin color. Towards this goal, collecting skin tone annotations has enabled bias identification in facial recognition, image captioning, person detection, skin image analysis in dermatology, face reconstruction and detecting deep fakes, among other tasks. In this paper, we build on this line of work and propose complementary and novel tools for measuring and extracting multidimensional skin color scores, and showcase their relevance and effectiveness for revealing dataset and model biases.
Changing the State of the Art - The Hue Angle
To demonstrate the relevance and benefits of a multidimensional skin color scale for fairness assessments in computer vision, first, we introduce a step towards more comprehensive apparent skin color scores. Rather than classifying skin color in types, as done with the Fitzpatrick scale, we measure automatically and quantitatively skin color in a multidimensional manner in images.
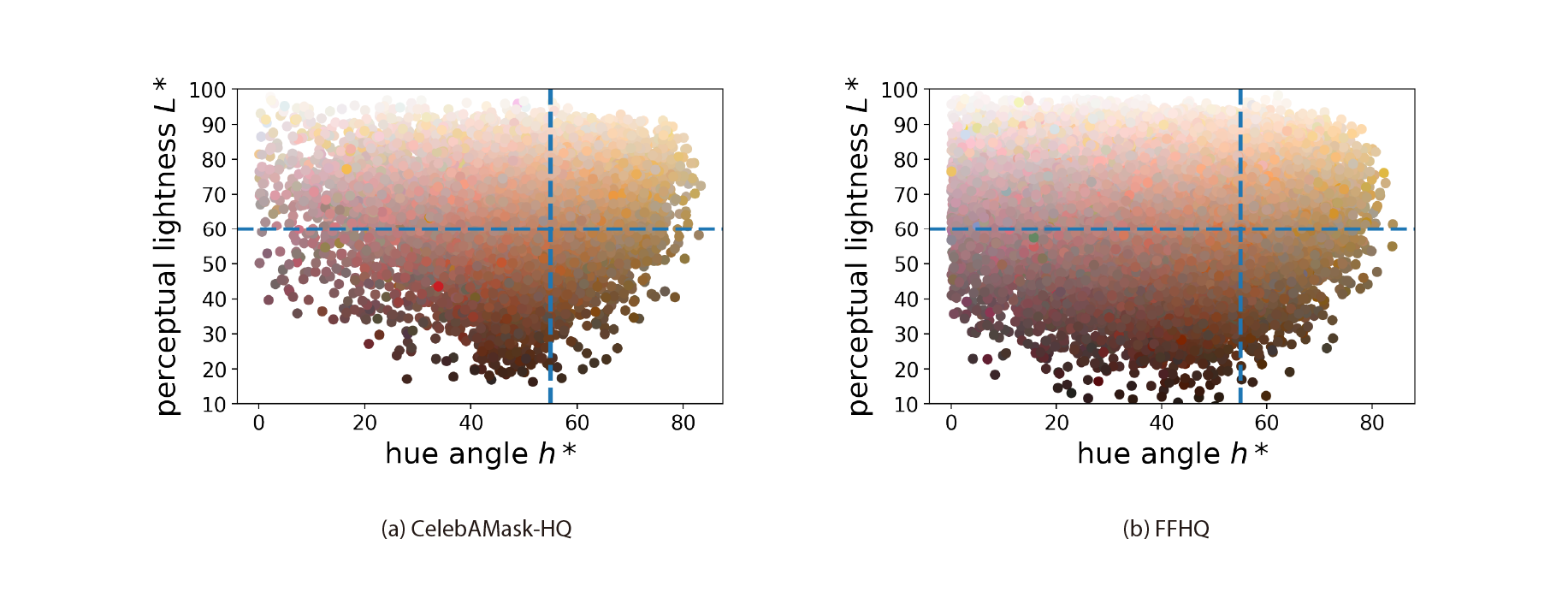
Figure 2: Skin color distribution on common face datasets. Every dot in the scatter plot corresponds to an im- age sample in the dataset. The skin tone threshold is at value 60 (light vs. dark), and the hue threshold at value 55◦ (red vs. yellow).
We then showcase the benefits of a multidimensional measure of skin color by quantifying to what extent common image datasets are skewed towards light-red skin color and under-represent dark-yellow skin color, and how generative models trained on these datasets reproduce a similar bias. This revealed multidimensional skin color biases in saliency-based image cropping and face verification models and the causal effect of skin color in attribute prediction in multiple commercial and non-commercial models. The results of assessing skin color in a multidimensional manner offer novel insights, previously invisible, to better understand biases in the fairness assessment of both datasets and models.
Our skin color scores inform which samples AI models are struggling with, and provide a solution by augmenting images or mitigating model representations in a multidimensional manner. By applying scientific approaches to color identification we utilized automation to overcome social constructs. Skin color was computed from a point measurement rather than risk the subjective nature of self-annotation.
A Multidimensional Approach Towards Fairness
Our approach was to first quantify the skin color bias in face datasets, and in generative models trained on such datasets. This reveals a skewness towards light-red skins and an under-representation of dark-yellow skins. Then we break down results by skin color of saliency-based image cropping and face verification algorithms. This reveals that model bias not only exists for skin tone, but also for skin hue.
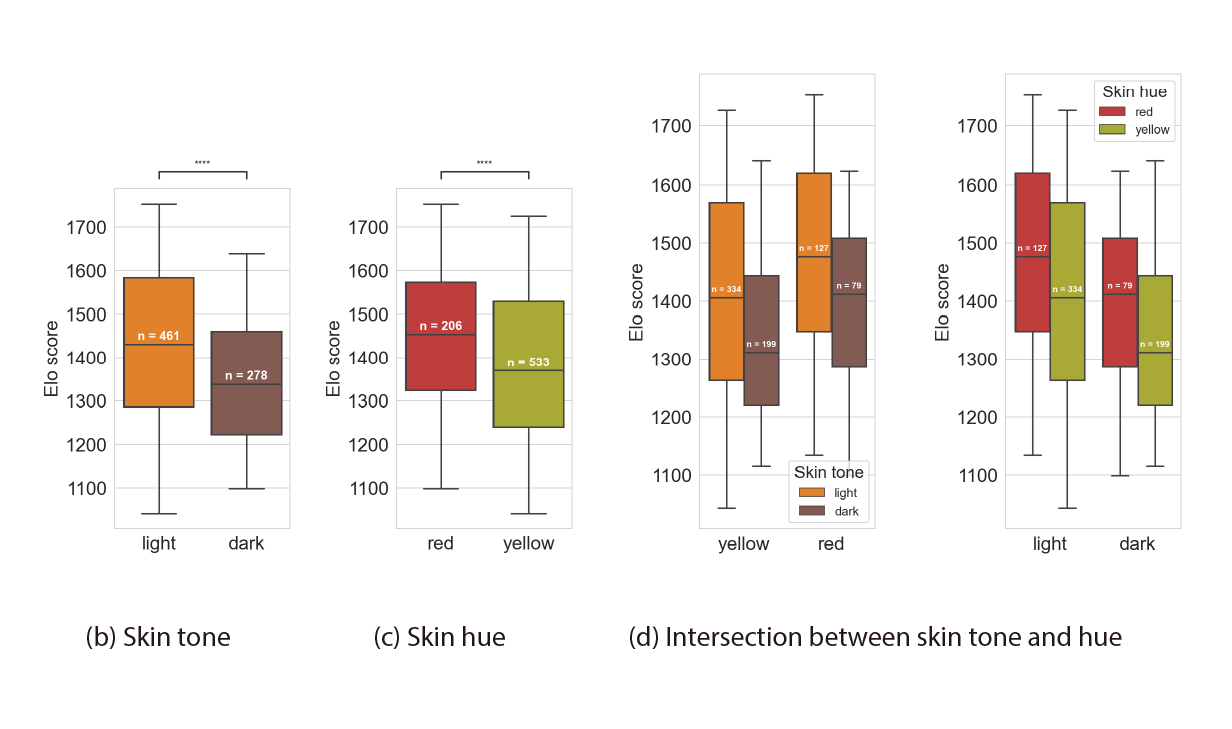
Figure 3: Saliency-based image cropping on CFD. (b) Performance differences between light and dark skin tones are statistically significant (p < 0.0001). (c) Differences between red and yellow skin hues are also statistically significant (p < 0.0001). (d) The intersectional analysis also reveals statistically significant differences (p < 0.01), except between light- yellow and dark-red skin colors. Complementary to the skin tone, the skin hue reveals additional differences in performance.
When we investigate the causal effect of skin color in attribute prediction, this reveals performance differences when skin color changes, as classifiers tend to predict people with lighter skin tones as more feminine, and people with redder skin hue as more smiley.
“We found quite plainly that people with a light red skin tone will tend to have a higher performance or be favored in the algorithm and people with a dark yellow skin will have a lower performance,” continued Thong. “This showed the wide discrepancy that exists among different demographic groups in terms of skin color.”
We observed that manipulating the skin color to have a lighter skin tone or a redder skin hue decreases the accuracy in non-smiling individuals as they tend to be predicted as smiling. Conversely, a darker skin tone or a yellower skin hue decreases the accuracy in smiling individuals. Overall, this benchmark reveals a bias towards a light skin tone when predicting if the individual belongs to the female gender, and a bias towards light or red skin hue when predicting the presence of a smile, which illustrates the importance of a multidimensional measure of skin color.
“That means there's a lot of bias that's not actually being detected, it's really important that we do not just think about diversity in this very black and white or light and dark way, but that we think of it as expansively and as globally as possible,” commented Xiang.
Moving Closer to True Skin Tone Bias Mitigation
There are two primary usages of this tool to train and deploy AI datasets and models, including generative AI models such as the StyleGAN and diffusion model for faces used in the research. Of course, to train an AI model requires the collection of a huge dataset. This tool can change how that data is tested for bias and inform researchers and developers in the field on how diverse the dataset is and identify if there's a particular group missing or that is underrepresented. With skin tone estimation, we have created a way to quantify the depth of the bias issue so that the data practitioner can decide to collect more data and ensure a more diverse dataset.
For existing datasets this new tool will provide an assessment that allows users to decide if the data set meets standards for diversity and bias mitigation and whether it should be used to train their models. While these use cases are more post-hoc, there is a potential to integrate our method at the start of AI projects when collecting human-centered images such that practitioners can monitor the skin color diversity during data collection.
While the paper considers face-related tasks, assessing skin color could also be applied to other human-centric tasks (e.g., pose estimation, segmentation, etc). This would help to enhance the diversity in the data collection process, by encouraging specifications to better represent skin color variability and improve the identification of dataset and model biases in fairness benchmarking, by highlighting their limitations and leading to fairness-aware training methods. We encourage the usage of a multidimensional skin color measure as a fairness tool to assess the computer vision pipeline, from data collection to model deployment.
By testing whether a dataset or an existing model is prone towards a specific skin color this tool acts as a mitigation technique to better train generative AI so that it can be more diverse in terms of generated output and move towards better representation of true human skin color variation. This multidimensional scale can now be used in place of the one-dimensional scales now in place for a better way to test for skin tone bias, moving beyond thinking about bias in one dimension.
We Strive for AI Systems That Are Not Only Powerful but Also Just and Unbiased.
Measuring apparent skin color requires a multidimensional score to capture its variation and provide a comprehensive representation of its constitutive complexity. This completely new approach to skin tone annotations and assessment serves as a simple, yet effective, first step towards a multidimensional skin color score in AI.
It's important that metrics like skin tone are captured more effectively because so many regard skin tone as far less sensitive than categories like race. That is why there is such a strong reliance on it, but also why it doesn't make sense to continue to use skin tone scales that were not developed for this purpose and are not designed for trying to capture global diversity and skin tone.
By revealing biases related to skin color in image datasets and computer vision models that were previously invisible, our multidimensional skin color scale offers a more representative assessment to surface socially relevant biases due to skin color effects in computer vision.
This builds on a growing corpus of work that examines ethical data collection and fairness benchmarking. This skin tone scale is an important first step towards enabling an additional dimension of bias to consider, measure and evaluate. We will continue to create additional tools and metrics to measure bias, collect effective benchmarks and consider the ethical dimensions to collecting sensitive data. By mitigating bias, enhancing transparency, and ensuring that we engage stakeholders in our AI research and development, Sony AI continues to be a leader in responsible AI development.
Read the full paper here:
https://ai.sony/publications/Beyond-Skin-Tone-A-Multidimensiona-Measure-of-Apparent-Skin-Color/
[1] Joy Buolamwini and Timnit Gebru. Gender shades: Inter- sectional accuracy disparities in commercial gender classifi- cation. In FAccT, 2018.
Latest Blog
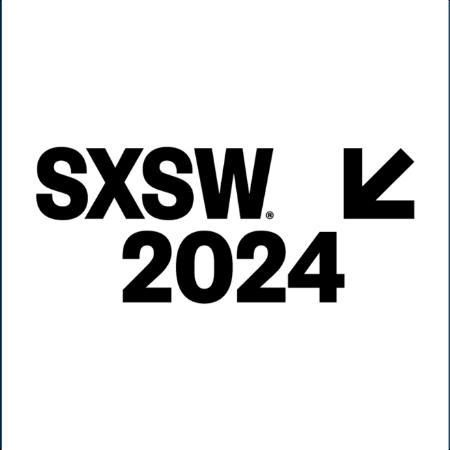
April 3, 2024 | Events
That’s A Wrap: Sony AI Ends Fiscal Year 2023 with SXSW, Two New Published Articl…
The past year marked a period of remarkable creativity, innovation, and excitement for Sony AI as it advanced its mission to research and develop AI that augments, and works in har…
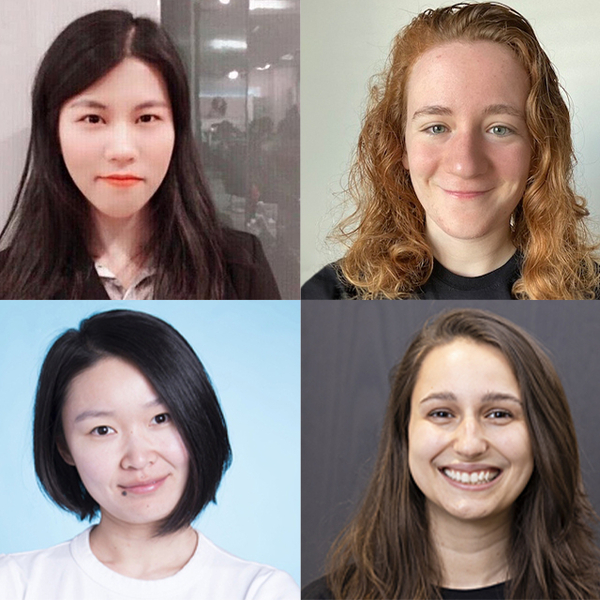
March 29, 2024 | Life at Sony AI
Celebrating the Women of Sony AI: Sharing Insights, Inspiration, and Advice
In March, the world commemorates the accomplishments of women throughout history and celebrates those of today. The United States observes March as Women’s History Month, while man…
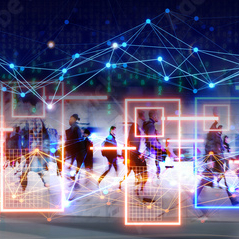
March 26, 2024 | AI Ethics
When Privacy and Fairness Collide: Reconciling the Tensions Between Privacy and …
Invisibility is sometimes thought of as a superpower. People often equate online privacy with selective invisibility, which sounds desirable because it potentially puts them in con…